Quantifying AI ROI: What Enterprises Need to Track (and What They Don't)
AI ROI isn't automatic. Adoption varies, metrics can mislead, and skill gaps remain. Track collaboration, onboarding, and real use, not just time saved. Move from isolated tools to integrated solutions. Focus on what truly drives business impact.
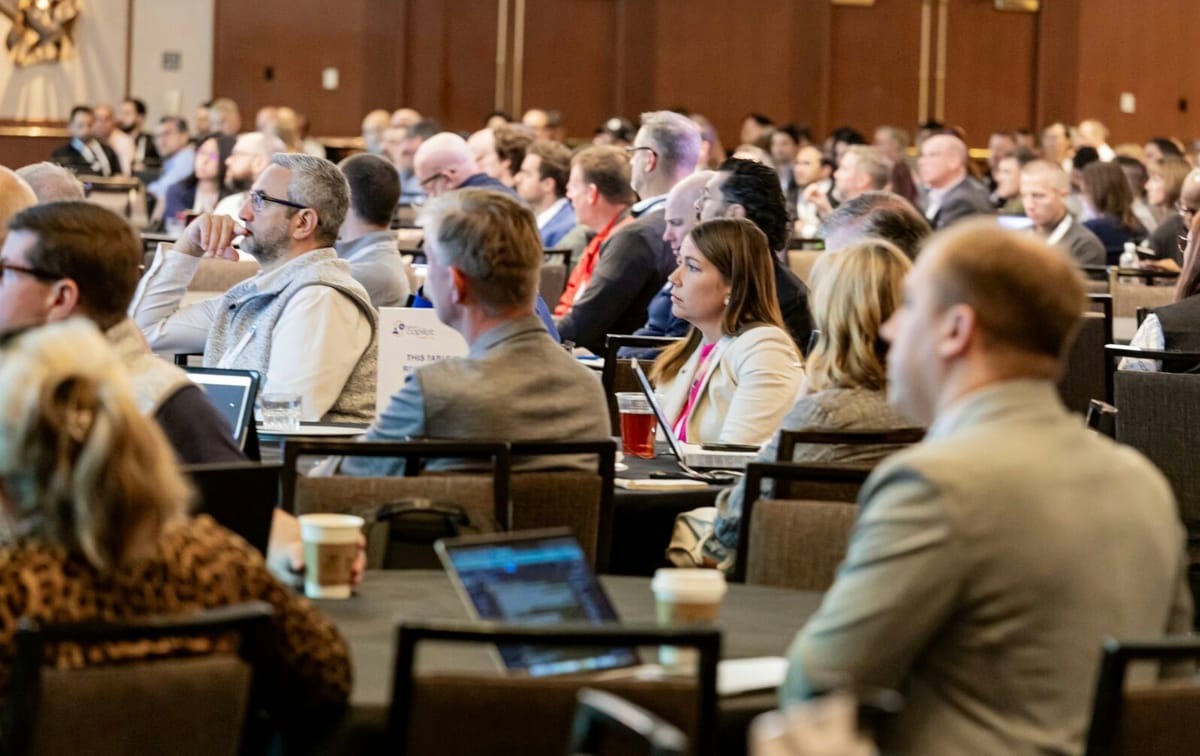
Enterprises face challenges in proving the effectiveness of AI tools despite adopting them successfully. Leaders may highlight a Copilot rollout or a successful automation pilot, but demonstrating the value remains tricky. A key takeaway at a recent AI Summit was that ROI tracking for AI needs improvement.
AI spending is increasing, but ROI metrics are lagging. It's not straightforward due to several reasons:
- Benefits from tools like Copilot or custom GPTs may not be immediate.
- Usage is uneven, with a small segment of users accounting for most activity.
- Evaluations are often isolated, missing cross-functional insights.
- Effective prompting is still not second nature to many users.
“ROI tracking for agents and assistants lags behind automation.”
A more realistic, business-aligned model is needed, focusing on adoption trends, skill gaps, cultural barriers, and cross-functional collaboration.
Adoption vs. Impact
Dashboards may look promising at first glance, but averages can be misleading. For example, Copilot data showed that:
- Only 12% of employees use it frequently (10+ actions per week).
- 24% of employees don't use it at all.
- Frequent users are primarily high-impact individual contributors rather than executives.
Success can't be claimed when usage is concentrated among a specific group. People may not share outputs due to fear, unclear policies, or job security concerns.
Measuring the Right Metrics
Organizations should focus on AI maturity rather than merely the number of tools purchased. It's about deep integration into the workflow. Key maturity stages include exploring, planning, implementing, scaling, and realizing AI.
Different stages come with varied ROI expectations, starting with productivity and later encompassing business growth.
Metrics to Track
Move beyond time savings and track:
- After-hours work reduction: Indicates better workload balance and well-being.
- Collaboration efficiency: Focus on quality over quantity in meetings and messages.
- 1:1 time with managers: Essential for onboarding and retention.
- Internal network size: A proxy for inclusion and connectivity.
- Onboarding completion and early tenure turnover: Linked to early-stage productivity and Copilot effectiveness.
This data is already available in tools like Viva Insights and should be tied to AI usage and investment.
From Projects to Portfolios
A common oversight is treating AI tools as isolated solutions. Instead, consider a portfolio approach:
- Set shared goals across teams.
- Integrate data across AI systems like Copilot, Viva, and Azure AI.
- Form AI councils that focus on impact rather than activity.
- Establish portfolio-level metrics instead of individual tool stats.
“You want to orchestrate AI tools into a harmonized portfolio after many have been independently implemented.”
This approach helps consolidate efforts and increase value.
People First, Not Just Prompts
Skill gaps remain a barrier, but the solution isn't just more training. Adopt smarter, social learning strategies:
- Use team nominations and not just individual champions.
- Encourage the sharing of AI results and use cases.
- Embed prompt best practices in workflows.
- Offer prompt libraries with explanations of their rationale.
Expect a temporary productivity dip before improvements emerge. Plan accordingly.
Conclusion
AI success depends on the ability to measure and evolve its use across the business, aligning with strategic goals rather than relying on default metrics. Building a measurement system that reflects business realities is crucial.
In the comments, share your experiences or challenges with AI. I'm interested in how you are addressing these issues.