Data Readiness for AI: What Enterprises Need to Get Right
AI success relies on quality data, yet poor data costs businesses trillions. A 2018 AI project failed due to bad data, while a 2024 one thrived by prioritizing data readiness. Key steps: align AI with goals, engage stakeholders, assess data, run pilots, and manage change.
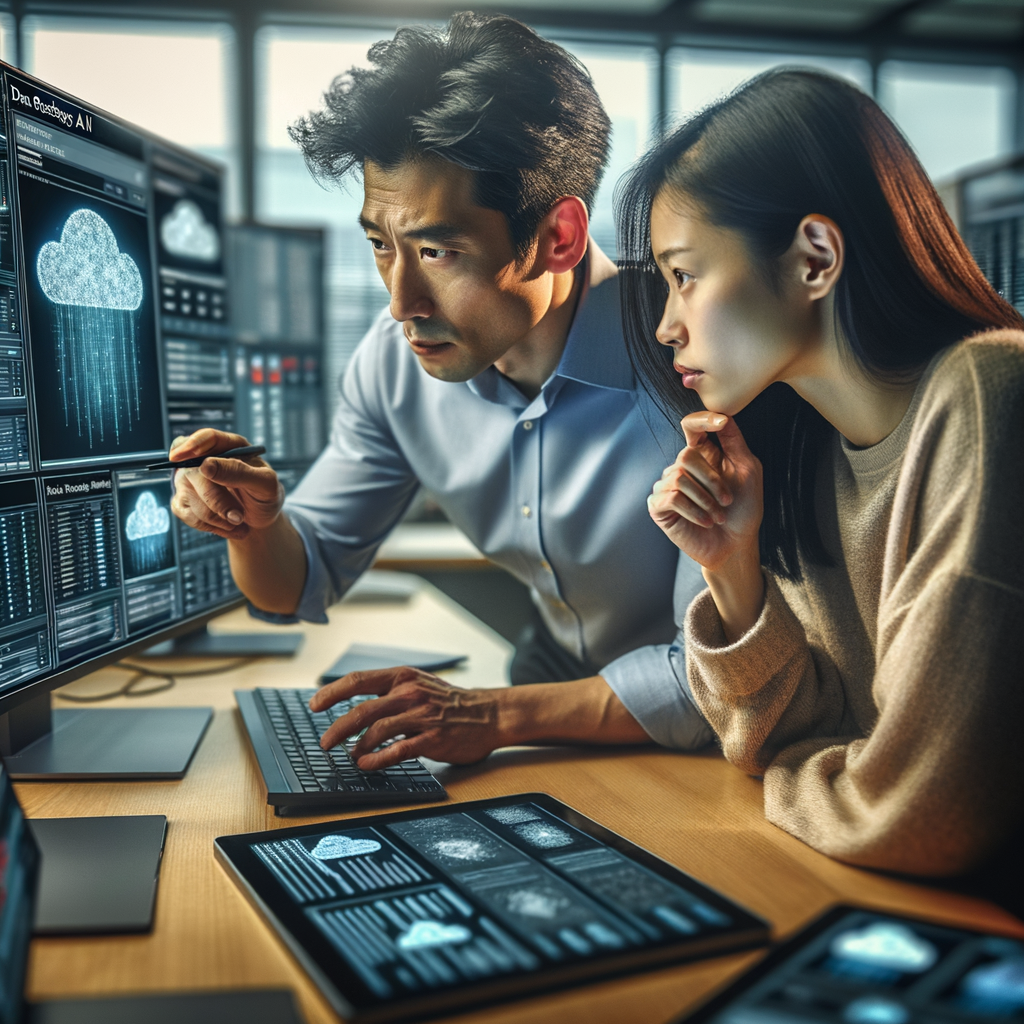
What if your AI project fails, not because of the technology, but because your data isn't ready?
That's the reality for many enterprises. AI depends on high-quality data. But 50% of employees' time is wasted handling low-value data tasks, and poor data quality costs U.S. businesses $3.1 trillion per year (MIT Sloan, IBM).
"Many AI projects fail not because of bad algorithms, but because they rely on bad data." — Michael G. Simms, Vice President of Data & AI at Columbus Global
A Tale of Two AI Projects: 2018 vs. 2024
A comparison of two real AI implementations shows how data readiness can make or break success.
2018: AI-Powered Food Sorting
A food distribution company launched a machine learning initiative to improve waste reduction and quality grading. The project had a strong technical team, but:
- Business SMEs were not involved from the start, leaving the AI model disconnected from real needs.
- Data quality was mixed, requiring extensive rework.
- Success metrics were unclear, making ROI difficult to measure.
The AI worked, but at a higher cost than expected.
2024: AI for Product Tagging in Apparel
A high-volume apparel company took a different approach with a large language model (LLM) for automated product tagging. The key differences:
- The project had two executive sponsors from both IT and the business.
- Data was assessed upfront to ensure accuracy and consistency.
- A proof of concept validated the approach before scaling.
The project delivered measurable business value instead of just proving an AI model could work.
How Enterprises Should Approach AI
Many AI initiatives start as tech-first projects, but successful ones begin with business needs. Here's what leaders need to focus on:
✅ Align AI with business strategy. AI must solve a high-impact problem, not just be an innovation experiment.
✅ Involve the right stakeholders. Business leaders, IT teams, and end users must collaborate from day one.
✅ Assess data quality first. Before launching AI, audit for accuracy, completeness, and bias in data.
✅ Start with a targeted proof of concept. A well-scoped pilot with clean, structured data reduces risks.
✅ Plan for change management. AI adoption isn't just about technology. Employees need to understand how their workflows will change.
"The wildcard in change and transformation is people. AI is a frightening topic. People are afraid of it, afraid of losing their value or job." — Community Summit NA 2024
The Role of Data Governance
A data governance framework is critical for AI success. This includes:
🔹 Data Quality Management to ensure clean, structured data for AI models.
🔹 Data Security & Compliance to meet regulations like GDPR and CCPA.
🔹 Bias Audits to prevent AI from reinforcing unfair or inaccurate patterns.
Why This Matters Now
Companies that fix their data issues before deploying AI gain a competitive advantage. Those that don't waste time and money on AI projects that never deliver value.
If your organization is exploring AI but facing data challenges, let's discuss how to build a data strategy that drives real business outcomes.